Unlocking Business Potential with Bayesian Statistical Analysis
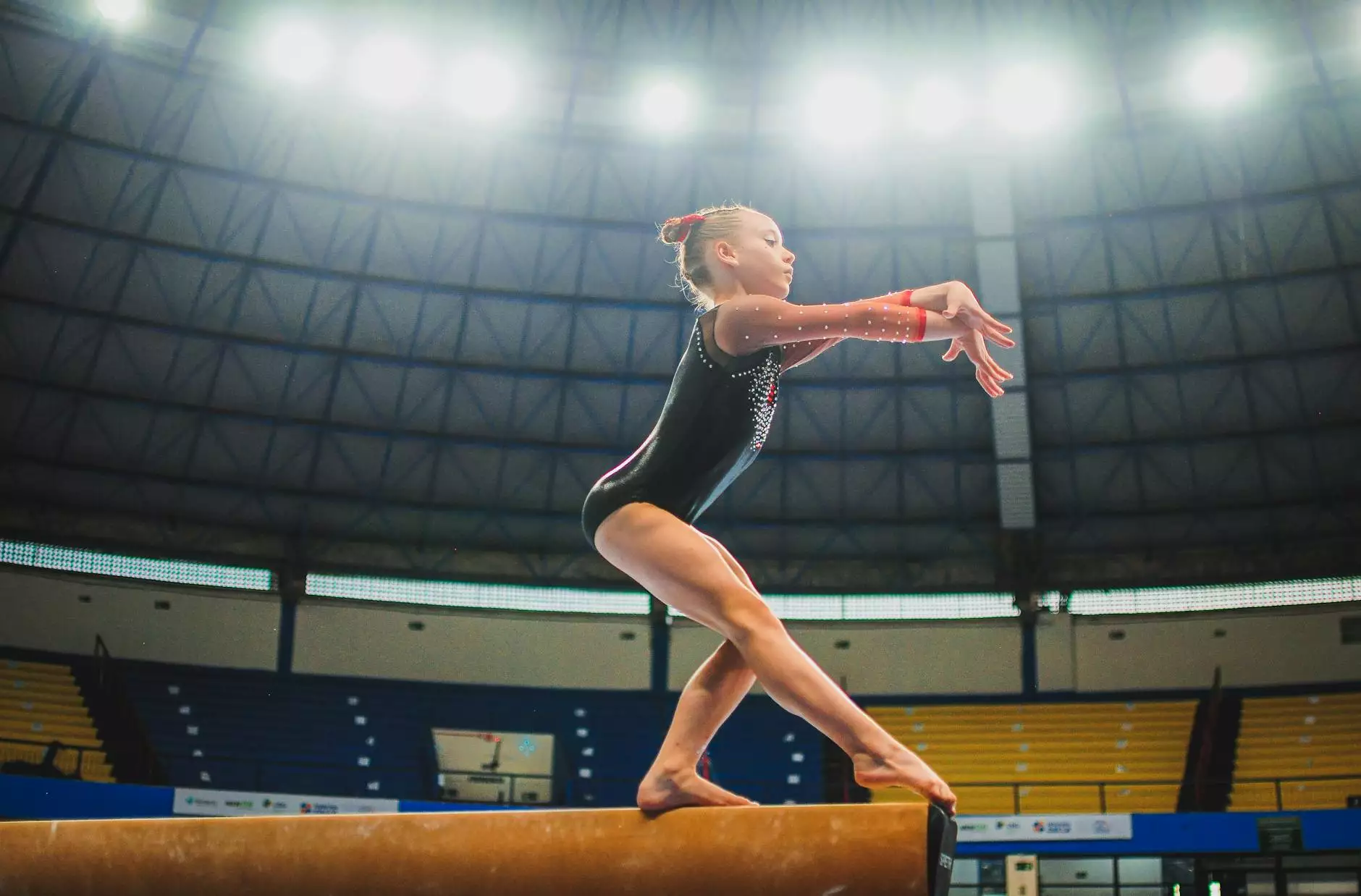
In the ever-evolving landscape of modern business, data-driven decision-making has emerged as a cornerstone for success. One powerful tool in this domain is Bayesian statistical analysis, a method that enables businesses to make informed decisions based on existing data while incorporating new information as it becomes available. This article delves into the core concepts of Bayesian statistical analysis and its applicability across various industries, particularly in IT Services & Computer Repair and Security Systems. By understanding and effectively implementing Bayesian analysis, businesses can enhance their strategic approaches, improve operational efficiencies, and ultimately drive profitability.
Understanding Bayesian Statistical Analysis
At its core, Bayesian statistical analysis is rooted in Bayes' Theorem, which describes how to update the probability for a hypothesis as evidence is acquired. It provides a mathematical framework for combining prior knowledge with new evidence to arrive at more refined estimates. Unlike traditional frequentist statistics, which rely heavily on fixed sample sizes and emphasize long-run frequencies, Bayesian methods allow for the integration of subjective belief and empirical data.
Key Components of Bayesian Analysis
- Prior Distribution: Represents the initial beliefs about a parameter before observing any data. This could stem from historical data, expert opinions, or any relevant information.
- Likelihood Function: Captures the likelihood of observing the data given a specific value of the parameter. This component is crucial as it determines how strongly the data supports the hypothesis.
- Posterior Distribution: The updated belief about the parameter after taking into account the prior distribution and the likelihood function. This is obtained using Bayes' theorem and represents the final assessment after observing the data.
Mathematical Formulation
Bayes' Theorem can be mathematically represented as follows:
P(H|E) = (P(E|H) * P(H)) / P(E)Where:
- P(H|E): Posterior probability - the probability of hypothesis H given evidence E.
- P(E|H): Likelihood - the probability of evidence E given hypothesis H.
- P(H): Prior probability - the initial probability of hypothesis H.
- P(E): Total probability of evidence E.
The Value of Bayesian Analysis in Business Decisions
Bayesian statistical analysis is revolutionizing how businesses approach decision-making. Its ability to incorporate prior knowledge with new data offers several advantages:
1. Enhanced Predictive Accuracy
In the realm of IT services and computer repair, accurately predicting equipment failures can save businesses significant time and resources. By employing Bayesian analysis, companies can analyze historical data on equipment performance and incorporate this information into predictive maintenance schedules. This leads to fewer unexpected failures and reduced downtime, ultimately improving customer satisfaction and retention.
2. Improved Risk Management
In the field of security systems, businesses face numerous uncertainties, from potential breaches to equipment efficacy. Bayesian methods allow companies to calculate the probabilities of various risks based on both historical data and ongoing assessments. This continuous updating of probabilities aligns internal security measures with real-time threats, ensuring a proactive stance on potential vulnerabilities.
3. Dynamic Decision-Making
Today's market demands agility. Through Bayesian analysis, decision-makers can adapt strategies on-the-fly by reevaluating probabilities as new data becomes available. For instance, when launching a new IT service, if early adoption metrics are lower than expected, Bayesian models can help determine if this trend will continue or if it represents a temporary anomaly. This adaptability is crucial for maintaining competitive advantage in rapidly changing industries.
Implementing Bayesian Analysis in Business Functions
To reap the benefits of Bayesian statistical analysis, businesses can integrate it into various functions:
1. Marketing Analytics
Marketing departments can utilize Bayesian methods to improve customer segmentation and targeting. By analyzing past campaign data, businesses can develop models that adapt as they gather more insights from ongoing campaigns, leading to optimized advertising spend and higher conversion rates.
2. Supply Chain Optimization
Bayesian analysis can significantly enhance supply chain operations by allowing businesses to forecast demand with greater accuracy. By continuously updating forecasts based on sales data and market trends, companies can better manage inventory levels, reduce costs, and improve customer service through timely deliveries.
3. Behavioral Analysis
Understanding customer behavior is a critical aspect of business success. Bayesian techniques enable businesses to analyze user interaction data from websites and applications, leading to more profound insights into customer preferences and behavior patterns. As a result, companies can refine their products and services to meet their customers' evolving needs.
Case Studies: Bayesian Analysis in Action
Case Study 1: IT Services Firm Prioritizing Customer Support
An IT services company implemented Bayesian analysis to prioritize customer support requests based on urgency and probability of escalations. By analyzing historical data related to service requests and customer interactions, the company developed a predictive model that improved response times and service quality. Consequently, customer satisfaction ratings rose by 25% within a year, emphasizing the power of data-driven decisions in operational efficiency.
Case Study 2: Security Systems Provider Enhancing Threat Response
A security systems provider utilized Bayesian analysis to enhance its threat detection capabilities. By continuously updating the likelihood of security threats based on emerging trends and previous incidents, the company improved its response times to breaches. This proactive stance led to a significant reduction in incidents, making their systems more reliable and trustworthy among clients.
Challenges and Considerations for Bayesian Analysis
While Bayesian statistical analysis offers numerous advantages, businesses must also be aware of its challenges:
1. Data Quality and Availability
Bayesian analysis relies heavily on the availability of high-quality, relevant data. If historical data is sparse or of poor quality, the validity of the analysis may be compromised. It is essential for businesses to invest in data collection and management processes to ensure that they can leverage Bayesian techniques effectively.
2. Complexity and Expertise
Implementing Bayesian analysis can be complex, requiring a solid understanding of statistics and modeling techniques. Businesses may need to invest in training existing staff or hiring experts familiar with Bayesian methods. However, the payoff in terms of improved decision-making can outweigh these initial costs.
3. Misinterpretation of Results
There’s a risk of misinterpreting the results generated by Bayesian analysis. Decision-makers should be aware of the assumptions underlying the models and ensure that they correctly understand and communicate these results to avoid misguided decisions.
Conclusion: The Future of Business with Bayesian Statistical Analysis
As businesses face increasingly complex environments characterized by rapid technological advancements and evolving consumer behaviors, the need for robust analytical frameworks has never been greater. Bayesian statistical analysis provides a powerful tool that can transform how organizations make decisions, enhancing accuracy, agility, and resilience.
By adopting Bayesian methods, particularly in crucial sectors like IT services and security systems, businesses can unlock new pathways to success. As organizations continue to generate vast amounts of data, integrating Bayesian analysis into their operations is not just a possibility; it is becoming a necessity for those looking to maintain a competitive edge in the global market.
In summary, by understanding and implementing Bayesian statistical analysis, businesses can not only improve their decision-making processes but also prepare themselves for the future by being equipped to harness the power of data more effectively.